Maytal Saar-Tsechansky
Professor
Department: Information, Risk & Operations Management
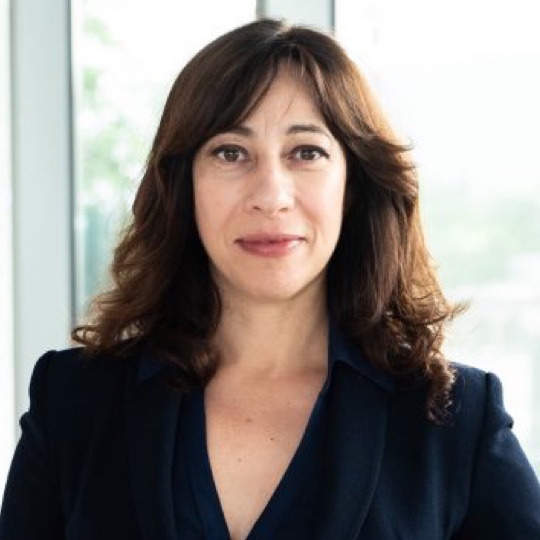
ACADEMIC LEADERSHIP & AWARDS
2022-23 |
Kleberg Foundation Grant, $500,000. |
2010 |
Chevron Centennial Fellowship in Business |
2006 |
MBA Honor Roll Award for Outstanding MBA Class Instruction, McCombs School of Business, Spring |
2006 |
National Science Foundation award: “Active Learning System for Audit Selection,” |
2006-07 |
Research Grant, The University of Texas at Austin |
2006-07 |
Research Excellence Grant, McCombs School of Business |
Mathias Kraus, Stefan Feuerriegel, and Maytal Saar-Tsechansky. Jan/Feb 2024. Data-Driven Allocation of Preventive Care with Application to Diabetes Mellitus Type II. Manufacturing & Service Operations Management 26(1): 137-153
Huimin Xu, Maytal Saar-Tsechansky, Min Song, and Ying Ding. Oct 2023.
Using Explainable AI to Understand Team Formation and Team Impact.
Proceedings of the Association for Information Science and Technology 60(1): 469-478.
Yunyi Li, Maria De-Arteaga, and Maytal Saar-Tsechansky. When More Data Lead Us Astray: Active Data Acquisition in the Presence of Label Bias. Proceedings of the Tenth AAAI Conference on Human Computation and Crowdsourcing 10(1):133-146.
Thomas Zueger, Simon Schallmoser, Mathias Kraus, Maytal Saar-Tsechansky, Stefan Feuerriegel, and Christoph Stettler. Machine Learning for Predicting the Risk of Transition from Prediabetes to Diabetes. Diabetes Technology & Therapeutics. Forthcoming.
Maria De‐Arteaga, Stefan Feuerriegel, and Maytal Saar‐Tsechansky. Oct 2022. Algorithmic Fairness in Business Analytics: Directions for Research and Practice. Production & Operations Management 31(10): 3749-3770.
Maytal Saar-Tsechansky, Tomer Geva, and Harel Lustiger. More for Less: Adaptive Labeling Payments in Online Labor Markets. Data Mining and Knowledge Discovery, forthcoming.
Tomer Giva and Maytal Saar-Tsechansky. 2021. Who Is a Better Decision Maker? Data-Driven Expert Ranking Under Unobserved Quality. Production and Operations Management 30(1), 127-144.
Elad Liebman, Maytal Saar-Tsechansky, and Peter Stone. 2019. The Right Music at the Right Time: Adaptive Personalized Playlists Based on Sequence Modeling. MIS Quarterly 43(3), 765-A6.
Hilah Geva, Gal Oestreicher-Singer, and Maytal Saar-Tsechansky. 2019. Using Retweets When Shaping Our Online Persona: Topic Modeling Approach. MIS Quarterly 43(2), 501-524.
Markus Peters, Maytal Saar-Tsechansky, Wolfgang Ketter, Sinead Williamson, Perry Groot, and Tom Heskes. 2018. A Scalable Preference Model for Autonomous Decision-Making. Machine Learning 107(6), 1039-1068.
Wolfgang Ketter, John E. Collins, and Maytal Saar-Tsechansky. 2018. Information Systems for a Smart Electricity Grid: Emerging Challenges and Opportunities. ACM Transactions on Management Information Systems 9(3), 1-22.
Meghana Deodhar, Joydeep Ghosh, Maytal Saar-Tsechansky, and Vineet Keshari. 2017. Active Learning with Multiple Localized Regression Models. INFORMS Journal on Computing 29(3), 503-522.
Maytal Saar-Tsechansky. 2015. The Business of Business Data Science in IS Journals. MIS Quarterly 39(4), iii-vi.
David Pardo, Peter Stone, Maytal Saar-Tsechansky, Tayfun Keskin, and Kerem Tomak. 2010. Adaptive Auction Mechanism Design and the Incorporation of Prior Knowledge. INFORMS Journal on Computing 22(3), 353-370.
Maytal Saar-Tsechansky, Prem Melville, and Foster Provost. 2009. Active Feature-Value Acquisition. Management Science 55, 664-684.
Gary Weiss, Bianca Zadrozny, and Maytal Saar-Tsechansky. 2008. Editorial: Special Issue on Utility Based Data Mining. Data Mining and Knowledge Discovery 17(2).
Maytal Saar-Tsechansky and Foster Provost. 2008. Handling Missing Values when Applying Classification Models. Journal of Machine Learning Research 9, 1625-1657.
Paul C Tetlock, Maytal Saar-Tsechansky, and Macskassy, Sofus. 2008. More Than Words: Quantifying Language to Measure Firms' Fundamentals. Journal of Finance 63, 1437-1467.
Provost, Foster, Melville, Prem, and Maytal Saar-Tsechansky. 2007. Data acquisition and cost-effective predictive modeling: targeting offers for electronic commerce, in The Proceedings of The Ninth International Conference on Electronic Commerce, Minneapolis, Minnesota.
Maytal Saar-Tsechansky and Provost, Foster. 2007. Decision-Centric Active Learning of Binary-Outcome Models. Information Systems Research 18, 4-22.
Pardoe, David, Sone, Peter, Maytal Saar-Tsechansky, and Tomak, Kerem. 2006. Adaptive Mechanism Design: A Metalearning Approach, in The Proceedings of The Eighth International Conference on Electronic Commerce,
Melville, Prem, Yang, Stewart M., Maytal Saar-Tsechansky, and Mooney, Raymond J. 2005. Active Learning for Probability Estimation using Jensen-Shannon Divergence, in The Proceedings of The 16th European Conference on Machine Learning (ECML), Porto, Portugal: ECML 2005.
Pardoe, David, Stone, Peter, Maytal Saar-Tsechansky, and Tomak, Kerem. 2005. Adaptive Auctions: Learning to Adjust to Bidders, in Workshop on Information Technologies and Systems (WITS), 2005.
Melville, P., Maytal Saar-Tsechansky, Provost, F., and Mooney, R.J.. 2005. An Expected Utility Approach to Active Feature-value Acquisition, in The Proceedings of the Fifth International Conference on Data Mining (ICDM-2005), Houston, TX.
Melville, P., Maytal Saar-Tsechansky, Provost, F., and Mooney, R.J.. 2005. Economical Active Feature-value Acquisition through Expected Utility Estimation, in Proceedings of the KDD-05 Workshop on Utility-Based Data Mining, Chicago, IL.
Maytal Saar-Tsechansky and Chen, Hsuan-Wei Michelle. 2005. Variance-Based Active Learning for Classifier Induction, in Workshop on Information Technologies and Systems (WITS), WITS 2005.
Melville, Prem, Maytal Saar-Tsechansky, Provost, Foster, and Mooney, Raymond J.. 2004. Active Feature Acquisition for Classifier Induction, in The Proceedings of The Fourth International Conference on Data Mining (ICDM-2004), Bighton, UK.
Maytal Saar-Tsechansky and Foster Provost. 2004. Active Sampling for Class Probability Estimation and Ranking. Machine Learning 54 (2), 153-178.
Maytal Saar-Tsechansky and Provost, Foster. 2001. Active Learning for Class Probability Estimation and Ranking, in Seventeenth International Joint Conference on Artificial Intelligence (IJCAI-01), Seattle, WA.
Maytal Saar-Tsechansky, Nava Pliskin, Gad Rabinowitz, and Mark Tsechansky. 2001. Monitoring Quality of Care with Relational Patterns. Topics in Health Information Management 22, 24-35.
Maytal Saar-Tsechansky, Nava Pliskin, Gad Rabinowitz, and Mark Tsechansky. 2001. Pattern Extraction for Monitoring Medical Practices, in Proceedings of the 34th Hawaii International Conference on Systems Sciences (HICSS), Maui, HI: IEEE Computer Society Press.
Maytal Saar-Tsechansky, Nava, Pliskin, Gadi, Rabinowitz, and Avi, Porath. 1999. Mining Relational Patterns from Multiple Relational Tables. Decision Support Systems 27, 177-195.